Essential Energy: Vegetation Monitoring
We improved the risk profiling of vegetation using remote geospatial sensing and AI, ensuring Essential Energy could provide uninterrupted electricity supply to millions across their network.
Challenge
To remotely detect hazardous vegetation, hence lowering the likelihood of trees falling onto powerlines and ensuring a consistent and uninterrupted supply of electricity throughout the entire network.
Approach
There are a number of factors to consider with respect to tree fall-in risk assessment, those being the health of the tree, the proximity to a power line, and the height of the vegetation (which is also typically enforced by government regulations).
When designing a remote asset management solution required to scan over 60,000sqkm across rural and urban landscapes, consideration must also be made for the robustness, scalability and update frequency of the solution—essentially, how often, how much and accurately can we monitor the network?
Typically, solutions have centred on ground surveillance—driving vehicles up and down power line corridors—and aerial flyovers—real-time human assessment of what can be seen from the cockpit or post-assessment via captured LiDAR and high-resolution RGB imagery.
These are certainly valid solutions for high-priority areas and should always be considered as a component of a larger solution, but lack considerably in terms of scaling the all-important spatial and temporal aspects of remote asset management.
In this context, earth observation via satellite imagery had always been hurdled by an inability to capture information pertaining to heights. However, we now have a solution for determining 3D information through generative AI. We built deep learning models trained on large-scale custom datasets consisting of coupled LiDAR and high-resolution satellite imagery.
Outcome
It took more than half a decade to capture LiDAR imagery across the majority of the network—now, we can generate height estimates across the entire network several times per year.
Thanks to the deep learning models created, we can now update a digital twin every time a new image is acquired. This speeds up the risk profiling process, leading to efficient improvements in remote asset management, all while cutting costs. By locating trees and other landmarks such as new buildings, roads, and water bodies in relation to power lines, we gain valuable insights that aid in risk profiling and maintenance scheduling.
Additionally, assessing vegetation health through vitality indices offers further valuable information in support of operational processes.
We’ve highlighted the use of generative deep learning models and satellite imagery for remote asset management to detect hazardous vegetation, assess tree fall-in risk and ensure uninterrupted electricity supply.
This project has emphasised the factors to consider in tree fall-in risk assessment and the limitations of traditional solutions such as ground surveillance and aerial flyovers.
The use of generative AI in determining 3D information from satellite imagery has led to significant efficiency improvements in remote asset management, with frequent updates, reduced costs, and valuable insights gained from locating trees and other landmarks in relation to power lines and assessing vegetation health through vitality indices.
Remote asset management is becoming increasingly mainstream not only in the energy sector, but in a plethora of other industries requiring similar and adjacent capability, in real estate, insurance, and mining, among others.
Case Studies
We have a strong reputation for taking on complex challenges. Find out more about the ground breaking work and projects we’ve undertaken.
- All
- Enterprise
- Defence
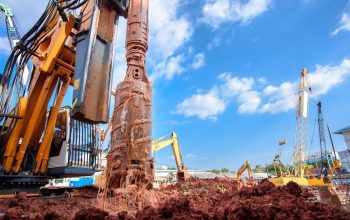
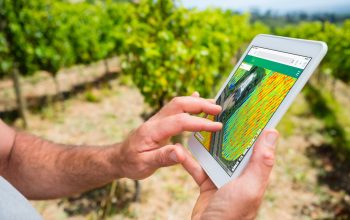


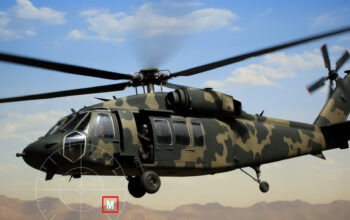

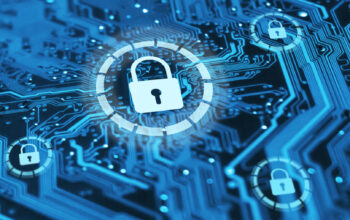



